GoShiken は C1000-185 試験「IBM watsonx Generative AI Engineer - Associate」のサンプル問題を無料で提供しています。購入する前、弊社の模擬試験画面や問題のクオリティー、使いやすさを事前に体験できます。
IBM watsonx Generative AI Engineer - Associate: C1000-185 試験
「IBM watsonx Generative AI Engineer - Associate」、C1000-185試験であります、IBM認定でございます。 最適な問題と解答をまとめられて、GoShiken はお客様のC1000-185試験に計 380 問をまとめてご用意いたしました。C1000-185試験の集結内容には、IBM Certified watsonx Generative AI Engineer - Associate認定にあるエリアとカテゴリの全てをカバーしており、お客様の IBM watsonx Generative AI Engineer - Associate 試験認定合格の準備を手助けをお届けします。
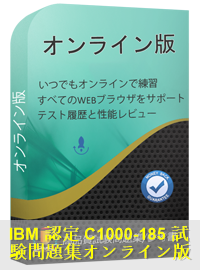
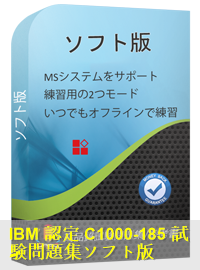
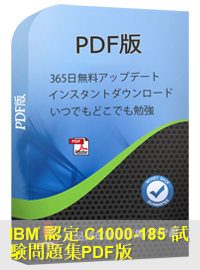
リアルなC1000-185テストエンジン
弊社のIBM watsonx Generative AI Engineer - Associate受験資料はお客様がIBM C1000-185試験を受けるために必要なすべてのものが含まれています。詳細はIBM watsonx Generative AI Engineer - Associate認証専門家側が研究して制作されて、彼らは業界の経験を利用して正確で論理的な制品を改良され続けています。
品質と価値のあるC1000-185試験問題
GoShiken練習試験IBM C1000-185は認定された対象分野の専門家と公開された作成者のみを招いて、最高水準の技術的精度で作成されています。
C1000-185試験合格を100%返金保証
お客様がもしGoShikenのテストエンジンを使って C1000-185 試験「IBM watsonx Generative AI Engineer - Associate」に不合格されました場合、弊社はお客様に購入金額を全額返金致します。
- C1000-185 試験に関する広範囲的な問題と解答
- C1000-185 試験問題集は事前使用できる
- 問題は業界の専門家によって調査されて、ほぼ100%正解率の検証済みの回答
- C1000-185 試験問題集は定期的に更新されます
- 本番試験を基づいてまとめられた C1000-185 問題集
- こちらの問題集は販売される前に複数回シミュレーション済み
- GoShiken で購入すると決める前に、無料で C1000-185 試験問題集のサンプルを試せます
365日無料アップデート
購入日から365日無料アップデートをご利用いただけます。365日後、C1000-185問題集更新版がほしく続けて50%の割引を与えれます。
インスタントダウンロード
お支払い後、弊社のシステムは、1分以内に購入したC1000-185問題集をあなたのメールボックスにお送りします。 2時間以内に届かない場合に、お問い合わせください。
100%返金保証
購入後60日以内に、C1000-185試験に合格しなかった場合は、全額返金します。 そして、無料で他の試験問題集を入手できます。
安全購入プロセス
弊社のC1000-185テスト連続は10年以上のビジネス経験の中で、お客様の購入権をひたすら重要視していますので、電子製品を購入する際にウイルスについて心配する必要はありません。 C1000-185試験準備の信頼性を長年にわたり評価され、保証された購入方法を提案するために限りなく努力を重ねるため、絶対的に安全な環境を構築しており、C1000-185試験問題にウイルスの攻撃されることはありません。 それについて疑いがある場合は、専門の担当者が最初にこれを処理します。また、C1000-185テストs問題セットをインストールして使用するために、リモートでオンラインで指導を受けることもできます。
すべての受験者にとって試験を簡単にするために、C1000-185試験対策ではテスト履歴の成績を確認することができます。その後、バツを見つけてそれを克服することができます。 さらに、この問題集のC1000-185試験問題をオンラインで一度利用した後は、次回オフライン環境で練習することもできます。 C1000-185テスト問題セットは、ユーザーが選択するためのさまざまな学習モードを提供いたします。これは、パソコンやスマホの複数登録ができてオンラインで勉強したり、オフラインで複数印刷するために使用できます。 値段がより安いため、より多くの選択肢が用意されています。試験のためにC1000-185試験問題を選択するのがオススメです。
提供された3つのバージョン
お客様にはさまざまなニーズを持っていることを考慮し、弊社は利用可能なC1000-185テスト問題セットを3つのバージョンで提供いたします--- PDF版、PCテストエンジン版とオンラインテストエンジン版。 最も有利なのが唯一サンプルを持つ--- Q&A形式のPDF版を無料でダウンロードできます。 こちらのバージョンはC1000-185試験準備に印刷可能で、ダウンロードすることが簡単にできます。つまり、いつでもどこでも勉強することができます。 PC版のC1000-185試験問題は実際の試験環境でリアル体験ができ、MSオペレーティングシステムをサポートします。これは試験のために勉強するためのより実用的な方法です。 さらに、C1000-185試験対策のオンラインテストエンジンは、ほとんどのユーザーがスマホ、タブレットでAPPを学習操作することに慣れているため、ほとんどの受験者の間で高い期待を寄せているようです。 それぞれのバージョンが同じ内容であることを保証します。お客様は自分が便利だと思うバージョンをお選びください。
IBM watsonx Generative AI Engineer - Associate 認定 C1000-185 試験問題:
1. You are implementing a Retrieval-Augmented Generation (RAG) system using IBM Watsonx to improve your generative AI model. The system retrieves relevant information from a large corpus and augments it into the generative process.
In this context, what role do embeddings play in a RAG-based system?
A) Embeddings store the entire content of documents, which is then directly passed to the generative model.
B) Embeddings reduce the size of the generative model by compressing the parameters into a smaller representation.
C) Embeddings ensure that only syntactically correct documents are retrieved, without regard to semantic content.
D) Embeddings are used to retrieve relevant documents by calculating the semantic similarity between user queries and the stored documents.
2. You are optimizing a generative AI model using prompts. You are tasked with choosing between a hard prompt and a soft prompt for generating a technical report.
Which option best describes a soft prompt in this context?
A) A prompt that directly instructs the model with domain-specific keywords and structured language to steer the model's output.
B) A prompt that restricts the model's output using hard-coded rules to ensure specific behavior during generation.
C) A prompt that influences the model by embedding learned vectors into the input, modifying the generation behavior indirectly.
D) A manually written prompt that is optimized using a grid search of the best keywords and patterns for the task.
3. A client has deployed a generative AI model to generate technical support responses for various issues in their product line. To improve the accuracy of the responses, they have been using tuned prompts for each type of technical issue (e.g., network issues, hardware failures). However, the client is concerned about occasional misclassifications when the prompts are reused across similar but distinct issues. They want to optimize the prompts to handle edge cases better without sacrificing the response speed.
What approach would be most appropriate for managing and optimizing these tuned prompts?
A) Create highly specific prompts for each possible issue, fine-tune the model on each prompt, and prioritize correctness over speed.
B) Use a single general prompt, apply greedy decoding, and manually edit outputs for any misclassifications.
C) Use a single tuned prompt for each product category, apply top-p sampling, and rely on post-processing to correct any misclassifications.
D) Leverage prompt chaining, where an initial general prompt narrows down the issue type, followed by a more specific tuned prompt for the final response.
4. You are tasked with building a Retrieval-Augmented Generation (RAG) system using Elasticsearch for document storage, Watson ML for model hosting, and LangChain for orchestration. The chatbot is supposed to query a large database of medical records and generate responses based on the retrieved information. During testing, you notice that irrelevant documents are often retrieved, leading to low-quality responses.
What would be the best approach to improve document relevance in this RAG setup?
A) Update the Elasticsearch index by re-indexing documents using embeddings from the Watson ML model.
B) Implement a BM25 similarity algorithm in Elasticsearch to improve document retrieval.
C) Fine-tune the Watson ML model on a dataset containing both queries and relevant documents, then update Elasticsearch with the model outputs.
D) Pre-process the medical records in LangChain to remove irrelevant information before sending the query to Elasticsearch.
5. During the deployment of a generative AI model using IBM Watsonx, you are responsible for ensuring that the model does not generate content containing hate speech, abusive language, or profanity. However, in some scenarios, the model might bypass basic filtering mechanisms and produce subtle or context-specific harmful language.
Which of the following best addresses edge cases where hate speech or abuse may slip through more basic filtering mechanisms?
A) Use sentiment analysis to detect and block negative content that may indicate hate speech or abuse.
B) Continuously monitor and retrain the model on datasets containing edge cases of hate speech, abuse, and profanity.
C) Use IBM Watsonx's HAP (Hate, Abuse, and Profanity) filter, which is designed to handle context-specific and subtle forms of harmful language.
D) Implement regular expression-based filters that cover a wide range of variations in harmful language.
質問と回答:
質問 # 1 正解: D | 質問 # 2 正解: C | 質問 # 3 正解: D | 質問 # 4 正解: A | 質問 # 5 正解: C |
6 お客様のコメント最新のコメント 「一部の類似なコメント・古いコメントは隠されています」
C1000-185の内容は問題数も増えた感じで内容も充実しているし、早速勉強していきたいと思います!
より効率良く合格を目指す私のための,必携のC1000-185試験対策書だと思う。理解しやすいC1000-185参考書だ。ありがとうございます
C1000-185内容は思った通り。とにかくやる気が起こるテキストです。
C1000-185使いやすかった。ここで感謝を申し上げます。ありがとうございました。
すごく効果的な問題集です。C1000-185試験に合格するために勉強資料を探す人にこの問題集を進めます。私は合格しました!誠にありがとうございます。
出た試験対策問題集は解き方がよくわかる詳しい解説が好きです。C1000-185参考書として、頼れる1冊です。